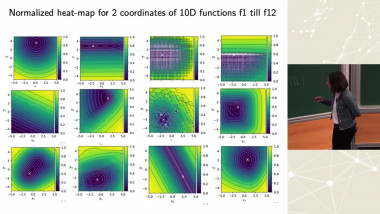

Slow Convergence of Stochastic Optimization Algorithms Without Derivatives Is Avoidable
By Anne Auger
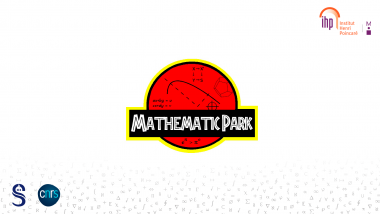

Le transport optimal en pratique : géométrie, algorithmes et applications
By Jean Feydy
Appears in collection : Schlumberger workshop - Computational and statistical trade-offs in learning
Renegar's condition number is a data-driven computational complexity measure for convex programs, generalizing classical condition numbers in linear systems. We provide evidence that for a broad class of compressed sensing problems, the worst case value of this algorithmic complexity measure taken over all signals matches the restricted eigenvalue of the observation matrix, which controls compressed sensing performance. This means that, in these problems, a single parameter directly controls computational complexity and recovery performance. Joint work with Vincent Roulet and Nicolas Boumal. Preprint: http://arxiv.org/abs/1506.03295