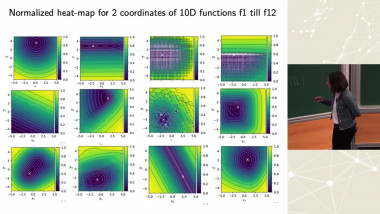

Slow Convergence of Stochastic Optimization Algorithms Without Derivatives Is Avoidable
By Anne Auger
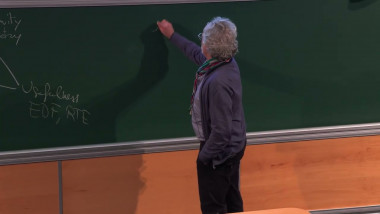

Deep Out-of-the-distribution Uncertainty Quantification in for Data (Science) Scientists
By Nicolas Vayatis
Appears in collection : Computational geometry days / Journées de géométrie algorithmique
In this talk, we investigate in a unified way the structural properties of a large class of convex regularizers for linear inverse problems. These penalty functionals are crucial to force the regularized solution to conform to some notion of simplicity/low complexity. Classical priors of this kind includes sparsity, piecewise regularity and low-rank. These are natural assumptions for many applications, ranging from medical imaging to machine learning. imaging - image processing - sparsity - convex optimization - inverse problem - super-resolution