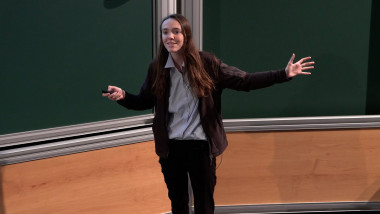

Deciding What Game to Play, What Mathematics Problem to Solve
By Katie Collins
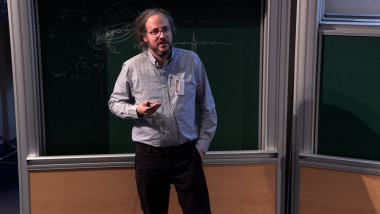

Machine Learning in PDE: Discovering New, Unstable Solutions
By Javier Gómez-Serrano
Appears in collection : SIGMA (Signal, Image, Geometry, Modeling, Approximation) / SIGMA (Signal, Image, Géométrie, Modélisation, Approximation)
we discuss classification problems in high dimension. We study classification problems using three classical notions: complexity of decision boundary, noise, and margin. We demonstrate that under suitable conditions on the decision boundary, classification problems can be very efficiently approximated, even in high dimensions. If a margin condition is assumed, then arbitrary fast approximation rates can be achieved, despite the problem being high-dimensional and discontinuous. We extend the approximation results ta learning results and show close ta optimal learning rates for empirical risk minimization in high dimensional classification.