

Climate Informatics: Machine Learning for the Study of Climate Change
By Claire Monteleoni


Impact and Legacy of the 2010 Paper on Self-force and EOB
By Leor Barack
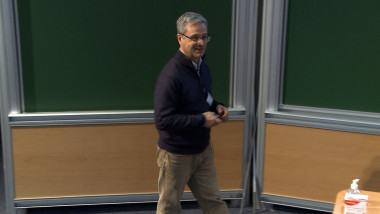

A Space Test of the Equivalence Principle with MICROSCOPE
By Manuel Rodrigues
By Gábor Drótos
Appears in collection : 2019 - T3 - WS1 - Nonlinear and stochastic methods in climate and geophysical fluid dynamics
The climate of a time instant under explicitly time-dependent forcing seems to be most plausibly defined by the natural probability measure of the corresponding snapshot attractor
(the instantaneous section of the full pullback attractor), and climate change is described by the time evolution of this probability measure.
This probability measure is numerically represented by an ensemble of trajectories (realizations) emanating from different initial conditions, but only after memory loss takes place. Recently, communities in Earth system modeling have started to develop initial-condition large ensembles' in state-of-the-art models. I will present a statistical analysis about the change of a correlation coefficient relating the El Nino-Southern Oscillation and the Indian monsoon in two of these large ensembles', and illustrate that the proper analysis may lead to conclusions qualitatively different from those relying on the time evolution of a single realization, questioning conclusions drawn about observations in the literature. After pointing out practical implications of the attractor framework (as compared to arbitrary analyses utilizing
initial-condition large ensembles'), I will discuss open questions about long time scales and temporal characteristics.