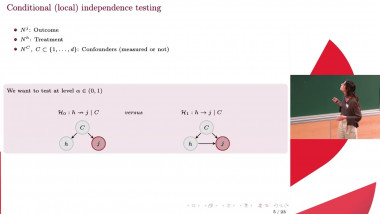

A Model for Testing Conditional Independence Applied to Event Processes
By Myrto Limnios


From robust tests to robust Bayes-like posterior distribution
By Yannick Baraud
By Julie Josse
Appears in collection : Mathematical Methods of Modern Statistics 2 / Méthodes mathématiques en statistiques modernes 2
Inferring causal effects of a treatment or policy from observational data is central to many applications. However, state-of-the-art methods for causal inference suffer when covariates have missing values, which is ubiquitous in application. Missing data greatly complicate causal analyses as they either require strong assumptions about the missing data generating mechanism or an adapted unconfoundedness hypothesis. In this talk, I will first provide a classification of existing methods according to the main underlying assumptions, which are based either on variants of the classical unconfoundedness assumption or relying on assumptions about the mechanism that generates the missing values. Then, I will present two recent contributions on this topic: (1) an extension of doubly robust estimators that allows handling of missing attributes, and (2) an approach to causal inference based on variational autoencoders adapted to incomplete data. I will illustrate the topic an an observational medical database which has heterogeneous data and a multilevel structure to assess the impact of the administration of a treatment on survival.