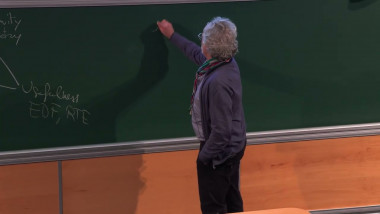

Deep Out-of-the-distribution Uncertainty Quantification in for Data (Science) Scientists
By Nicolas Vayatis
Appears in collection : New challenges in high-dimensional statistics / Statistique mathématique
Compared to artificial neural networks (ANNs), the brain seems to learn faster, generalize better to new situations and consumes much less energy. ANNs are motivated by the functioning of the brain but differ in several crucial aspects. While ANNs are deterministic, biological neural networks (BNNs) are stochastic. Moreover, it is biologically implausible that the learning of the brain is based on gradient descent. In the past years, statistical theory for artificial neural networks has been developed. The idea now is to extend this to biological neural networks, as the future of AI is likely to draw even more inspiration from biology. In this lecture series we will survey the challenges and present some first statistical risk bounds for different biologically inspired learning rules.