

Creative telescoping for D-finite functions - Lecture 1
By Christoph Koutschan


Creative telescoping for D-finite functions - Lecture 2
By Christoph Koutschan


Derandomization, tutorial - part 1: Pseudo-randomness from hardness
By Valentine Kabanets
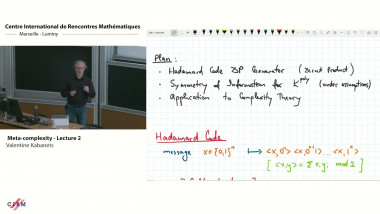

Derandomization, tutorial - part 2: Meta-complexity
By Valentine Kabanets