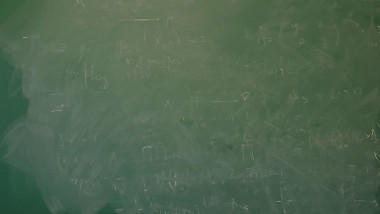

Exploring the High-dimensional Random Landscapes of Data Science (3/3)
By Gérard Ben Arous
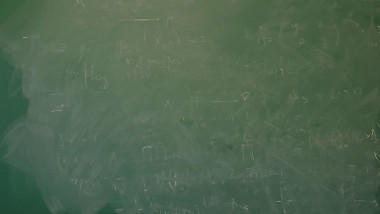

Generative AI and Diffusion Models: a Statistical Physics Analysis (2/3)
By Giulio Biroli
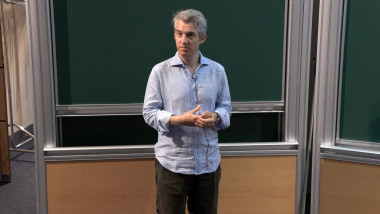

Generative AI and Diffusion Models: a Statistical Physics Analysis (1/3)
By Giulio Biroli
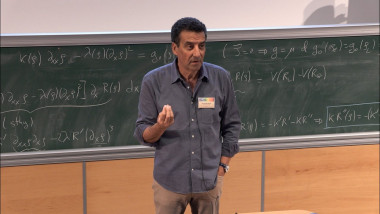

Exploring the High-dimensional Random Landscapes of Data Science (1/3)
By Gérard Ben Arous
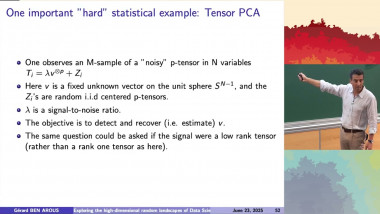

Exploring the High-dimensional Random Landscapes of Data Science (2/3)
By Gérard Ben Arous