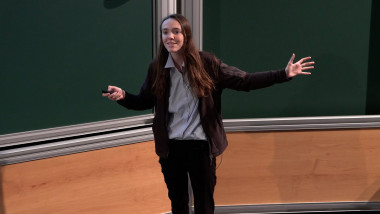

Deciding What Game to Play, What Mathematics Problem to Solve
By Katie Collins
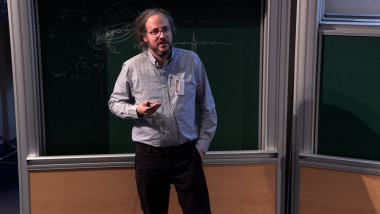

Machine Learning in PDE: Discovering New, Unstable Solutions
By Javier Gómez-Serrano
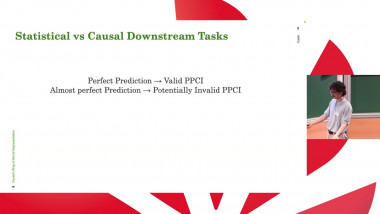

Causal Lifting of Neural Representations: Zero-Shot Generalization for Causal Inferences
By Riccardo Cadei
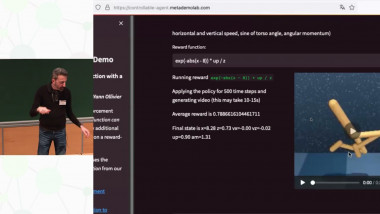

Universal Optimal Control, Reinforcement Learning, and Reaching Goals in LLMs
By Yann Ollivier