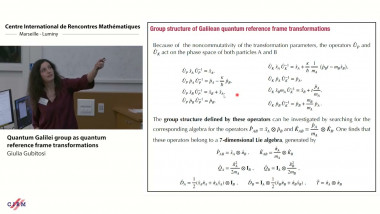

Quantum Galilei group as quantum reference frame transformations
De Giulia Gubitosi
Apparaît dans la collection : 2018 - T2 - WS1 - Observability and estimation in quantum dynamics
In quantum mechanics, measurement restores a classical notion of reality via collapse of the wavefunction, which yields a precisely defined outcome. On the other hand, the Heisenberg uncertainty principle dictates that incompatible observables, such as position and momentum, cannot both take on arbitrarily precise values. But how does a wavefunction evolve when two such quantities are probed simultaneously, and how does the uncertainty principle dynamically inhibit precise measurement outcomes? In the first part of this talk I will present a novel detection scheme that allows control over the measurement operators of multiple readout channels of a superconducting qubit. I will show how the uncertainty principle governs the quantum trajectory of the state by enforcing a lower bound on the measurement-induced disturbance, inhibiting wavefunction collapse and consequently leading to persistent diffusion [1]. In the second part, building on this experiment, I will show how machine learning models can be trained to predict quantum trajectories solely from the stream of experimental data without prior on quantum mechanics. In particular, we demonstrate that a recurrent neural network can be trained in real time to infer the quantum evolution of a superconducting qubit under non-trivial unitary evolution and continuous measurement from raw experimental observations only. This model independent prediction can be exploited to extract the system Hamiltonian, measurement operators and parameters such as quantum efficiency with a greater accuracy than usual calibration methods.